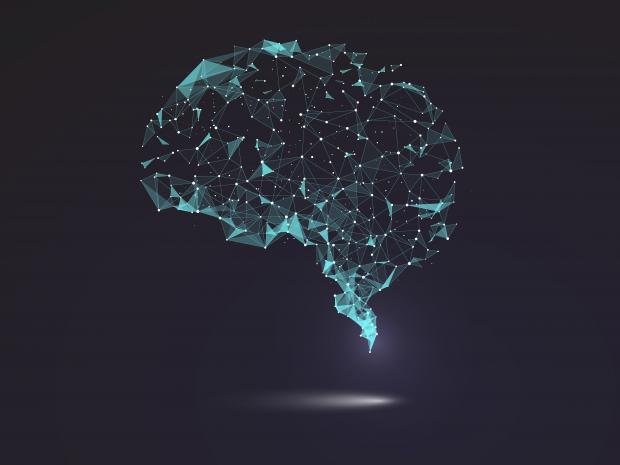
Part of the Special ECE Seminar Series
Modern Artificial Intelligence
Title:
Spectral Transformers
Speaker:
Elad Hazan, Princeton University
Abstract:
We'll discuss a new technique for sequence modeling for prediction tasks with long range dependencies and fast inference/generation. At the heart of the method is a new formulation for state space models (SSMs) based on learning linear dynamical systems with the spectral filtering algorithm. This gives rise to a novel sequence prediction architecture we call a spectral state space model. Spectral state space models have two primary advantages. First, they have provable robustness properties as their performance depends on neither the spectrum of the underlying dynamics nor the dimensionality of the problem. Second, these models are constructed with fixed convolutional filters that do not require learning while still outperforming SSMs in both theory and practice. The resulting models are evaluated on synthetic dynamical systems as well as long-range prediction tasks of various modalities. These evaluations support the theoretical benefits of spectral filtering for tasks requiring very long range memory. We will also discuss recent work showing fast generation and provable length generalization of these models.
More information about spectral transformers and filtering
Bio:
Elad Hazan is a professor of computer science at Princeton University. His research focuses on the design and analysis of algorithms for basic problems in machine learning and optimization. Among his contributions are the co-invention of the AdaGrad algorithm for deep learning, the first sublinear-time algorithms for convex optimization, and online nonstochastic control theory. He is the recipient of the Bell Labs Prize, the IBM Goldberg best paper award twice, a European Research Council grant, a Marie Curie fellowship and twice the Google Research Award. He served on the steering committee of the Association for Computational Learning and was program chair for the Conference on Learning Theory 2015. He is the co-founder and director of Google AI Princeton.