Chen Feng
-
Institute Associate Professor
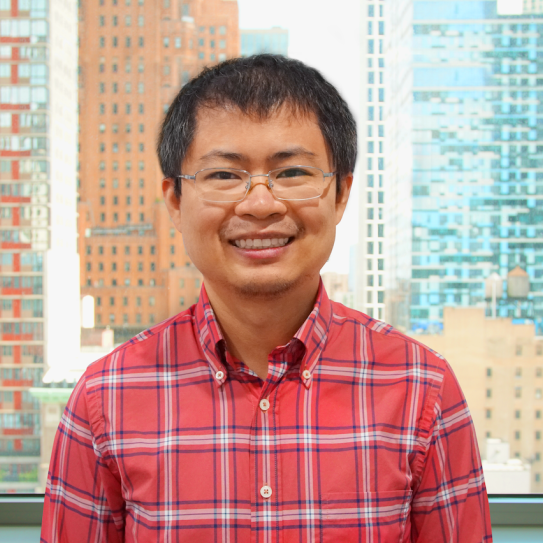
Chen Feng is an Associate Professor at NYU. He is interested in active and collaborative robot perception and learning through multidisciplinary use-inspired research that originates from construction, manufacturing, and transportation domains. Before NYU, Chen was a research scientist in the computer vision group at Mitsubishi Electric Research Labs (MERL) in Cambridge, MA, focusing on localization, mapping, and deep learning for self-driving cars and robotics, and invented several patented algorithms. Chen holds a bachelor’s degree in geospatial engineering from Wuhan University in China, a master’s degree in electrical engineering, and a Ph.D. in civil engineering, both from the University of Michigan at Ann Arbor. Chen publishes in and reviews for prestigious AI and Robotics venues like CVPR/ICCV/ICRA/IROS, and he also serves as an associate editor for IEEE Robotics and Automation Letters (RA-L). Chen is a recipient of the NSF CAREER Award in 2023. More information on his research can be found at https://ai4ce.github.io/.
Chen leads the AI4CE lab (pronounced as "A-I-force") with students from several departments (CSE, MAE, CUE, ECE) and schools (Tandon and Courant) at NYU. The AI4CE lab conducts multidisciplinary use-inspired research to develop novel algorithms and systems for intelligent agents to accurately understand and efficiently interact with materials and humans in dynamic and unstructured environments. We aim to fundamentally advance Robotics and AI in areas such as localization, mapping, navigation, mobile manipulation, and scene understanding, to address infrastructure challenges on Earth and beyond, including construction robotics, manufacturing automation, and autonomous vehicles.
Research News
AI-powered and Robot-assisted Manufacturing for Modular Construction
Semiha Ergan, assistant professor in the Departments of Civil and Urban Engineering, and Computer Science and Engineering and Chen Feng, assistant professor in the departments of Civil and Urban and Mechanical and Aerospace Engineering will lead this project.
Modular construction, with an established record of accelerating projects and reducing costs, is a revolutionary way to transform the construction industry. However, new construction capabilities are needed to perform modular construction at scale, where, as is the case in factories, the industry suffers from the dependency on skilled labor. Among the challenges this project aims to address:
- Every project is unique and requires efficiency and accuracy in recognition and handling workpieces
- Design and production-line changes are common, and require design standardization and optimization of modules, and
- Production lines are complex in space and time, and necessitate the guidance of workers while processing design and installation information accurately
To focus on these challenges while exploring modular construction within the context of Future Manufacturing (FM), this project exploits opportunities at the intersection of AI/robotics/building information modeling and manufacturing, with the potential to increase the scalability of modular construction.
The research will pioneer initial formulations to enable (a) high throughput in manufacturing through the definition and evaluation of processes that embrace real-time workpiece semantic grounding and in-situ AR-robotic assistance, (b) feasibility studies of optimizing and standardizing the design of modules, and utilization of a cyber-infrastructure for their standardization, (c) prototyping cyber-infrastructures as both novel ways of forming academia and industry partnerships, and data infrastructures to accelerate data-driven adaption in FM for modular construction, and (d) synergistic activities with a two-year institution to train and educate FM workforce for the potential of FM and technologies evaluated.
The team argues that, while the evaluations of technologies will focus on the modular construction, the proposed technologies could make manufacturing industries more competitive, particularly heavy manufacturing industries that share similar challenges such as agricultural, mining, and ship building. The project will therefore enhance U.S. competitiveness in production, bolster economic growth, educate students, and influence workforce behavior towards efficiency and accuracy with the skills required for leadership in FM.