Claudio Silva
-
Institute Professor
-
Professor of Computer Science
-
Professor of Data Science
-
Co-Director of the VIDA Center
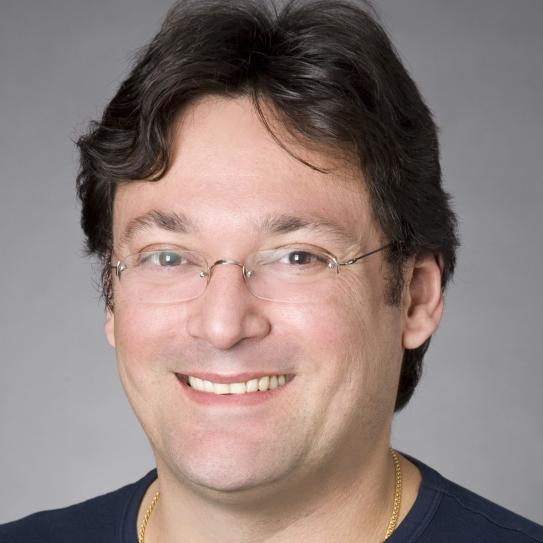
Cláudio T. Silva is Institute Professor of Computer Science and Engineering at NYU Tandon School of Engineering, and Professor of Data Science at the NYU Center for Data Science. He is also affiliated with the Center for Urban Science and Progress (which he helped co-found in 2012) and the Courant Institute of Mathematical Sciences. He received his BS in mathematics from the Universidade Federal do Ceará (Brazil) in 1990, and his MS and PhD in computer science at the State University of New York at Stony Brook in 1996. Claudio has advised 20+ PhD, 10 MS students, and mentored 20+ post-doctoral associates. He has published over 300 publications, including 20 that have received best paper awards. He has over 26,000 citations according to Google Scholar. Claudio is active in service to the research community and is a past elected Chair of the IEEE Technical Committee on Visualization and Computer Graphics (2015–18).
Claudio is a Fellow of the IEEE and has received the IEEE Visualization Technical Achievement Award. He was the senior technology consultant (2012-17) for MLB Advanced Media’s Statcast player tracking system, which received a 2018 Technology & Engineering Emmy Award from the National Academy of Television Arts & Sciences (NATAS). His work has been covered in The New York Times, The Economist, ESPN, and other major news media.
Education
Universidade Federal do Ceara 1990
BS, Mathematics
State University of New York at Stony Brook 1996
PhD, Computer Science
Experience
New York University School of Engineering
Professor
From: July 2011 to present
University of Utah
Professor
From: August 2003 to June 2011
Information for Mentees
About Me: I was Professor of Computer Science at the University of Utah (2003-2011) before coming to NYU in 2011. I am currently appointed between Tandon CSE and the Center for Data Science. At Tandon, I've served as Chair of TPC.
Research News
New tool helps analyze pilot performance and mental workload in augmented reality
In the high-stakes world of aviation, a pilot's ability to perform under stress can mean the difference between a safe flight and disaster. Comprehensive and precise training is crucial to equip pilots with the skills needed to handle these challenging situations.
Pilot trainers rely on augmented reality (AR) systems for teaching, by guiding pilots through various scenarios so they learn appropriate actions. But those systems work best when they are tailored to the mental states of the individual subject.
Enter HuBar, a novel visual analytics tool designed to summarize and compare task performance sessions in AR — such as AR-guided simulated flights — through the analysis of performer behavior and cognitive workload.
By providing deep insights into pilot behavior and mental states, HuBar enables researchers and trainers to identify patterns, pinpoint areas of difficulty, and optimize AR-assisted training programs for improved learning outcomes and real-world performance.
HuBar was developed by a research team from NYU Tandon School of Engineering that will present it at the 2024 IEEE Visualization and Visual Analytics Conference on October 17, 2024.
“While pilot training is one potential use case, HuBar isn't just for aviation,” explained Claudio Silva, NYU Tandon Institute Professor in the Computer Science and Engineering (CSE) Department, who led the research with collaboration from Northrop Grumman Corporation (NGC). “HuBar visualizes diverse data from AR-assisted tasks, and this comprehensive analysis leads to improved performance and learning outcomes across various complex scenarios.”
“HuBar could help improve training in surgery, military operations and industrial tasks,” said Silva, who is also the co-director of the Visualization and Data Analytics Research Center (VIDA) at NYU.
The team introduced HuBar in a paper that demonstrates its capabilities using aviation as a case study, analyzing data from multiple helicopter co-pilots in an AR flying simulation. The team also produced a video about the system.
Focusing on two pilot subjects, the system revealed striking differences: one subject maintained mostly optimal attention states with few errors, while the other experienced underload states and made frequent mistakes.
HuBar's detailed analysis, including video footage, showed the underperforming copilot often consulted a manual, indicating less task familiarity. Ultimately, HuBar can enable trainers to pinpoint specific areas where copilots struggle and understand why, providing insights to improve AR-assisted training programs.
What makes HuBar unique is its ability to analyze non-linear tasks where different step sequences can lead to success, while integrating and visualizing multiple streams of complex data simultaneously.
This includes brain activity (fNIRS), body movements (IMU), gaze tracking, task procedures, errors, and mental workload classifications. HuBar's comprehensive approach allows for a holistic analysis of performer behavior in AR-assisted tasks, enabling researchers and trainers to identify correlations between cognitive states, physical actions, and task performance across various task completion paths.
HuBar's interactive visualization system also facilitates comparison across different sessions and performers, making it possible to discern patterns and anomalies in complex, non-sequential procedures that might otherwise go unnoticed in traditional analysis methods.
"We can now see exactly when and why a person might become mentally overloaded or dangerously underloaded during a task," said Sonia Castelo, VIDA Research Engineer, Ph.D. student in VIDA, and the HuBar paper’s lead author. "This kind of detailed analysis has never been possible before across such a wide range of applications. It's like having X-ray vision into a person's mind and body during a task, delivering information to tailor AR assistance systems to meet the needs of an individual user.”
As AR systems – including headsets like Microsoft Hololens, Meta Quest and Apple Vision Pro – become more sophisticated and ubiquitous, tools like HuBar will be crucial for understanding how these technologies affect human performance and cognitive load.
"The next generation of AR training systems might adapt in real-time based on a user's mental state," said Joao Rulff, a Ph.D. student in VIDA who worked on the project. "HuBar is helping us understand exactly how that could work across diverse applications and complex task structures."
HuBar is part of the research Silva is pursuing under the Defense Advanced Research Projects Agency (DARPA) Perceptually-enabled Task Guidance (PTG) program. With the support of a $5 million DARPA contract, the NYU group aims to develop AI technologies to help people perform complex tasks while making these users more versatile by expanding their skillset — and more proficient by reducing their errors. The pilot data in this study came from NGC as part of the DARPA PTG
In addition to Silva, Castelo and Rulff, the paper’s authors are: Erin McGowan, PhD Researcher, VIDA; Guande Wu, Ph.D. student, VIDA; Iran R. Roman, Post-Doctoral Researcher, NYU Steinhardt; Roque López, Research Engineer, VIDA; Bea Steers, Research Engineer, NYU Steinhardt; Qi Sun, Assistant Professor of CSE, NYU; Juan Bello, Professor, NYU Tandon and NYU Steinhardt; Bradley Feest, Lead Data Scientist, Northrop Grumman Corporation; Michael Middleton, Applied AI Software Engineer and Researcher, Northrop Grumman Corporation, and PhD student, NYU Tandon; Ryan McKendrick, Applied Cognitive Scientist, Northrop Grumman Corporation.
arXiv:2407.12260 [cs.HC]
NYU Tandon researchers unveil tool to help developers create augmented reality task assistants
Augmented reality (AR) technology has long fascinated both the scientific community and the general public, remaining a staple of modern science fiction for decades.
In the pursuit of advanced AR assistants – ones that can guide people through intricate surgeries or everyday food preparation, for example – a research team from NYU Tandon School of Engineering has introduced Augmented Reality Guidance and User-Modeling System, or ARGUS.
An interactive visual analytics tool, ARGUS is engineered to support the development of intelligent AR assistants that can run on devices like Microsoft HoloLens 2 or MagicLeap. It enables developers to collect and analyze data, model how people perform tasks, and find and fix problems in the AR assistants they are building.
Claudio Silva, NYU Tandon Institute Professor of Computer Science and Engineering and Professor of Data Science at the NYU Center for Data Science, leads the research team that will present its paper on ARGUS at IEEE VIS 2023 on October 26, 2023, in Melbourne Australia. The paper received Honorable Mention in that event’s Best Paper Awards.
“Imagine you’re developing an AR AI assistant to help home cooks prepare meals,” said Silva. “Using ARGUS, a developer can monitor a cook working with the ingredients, so they can assess how well the AI is performing in understanding the environment and user actions. Also, how the system is providing relevant instructions and feedback to the user. It is meant to be used by developers of such AR systems.”
ARGUS works in two modes: online and offline.
The online mode is for real-time monitoring and debugging while an AR system is in use. It lets developers see what the AR system sees and how it's interpreting the environment and user actions. They can also adjust settings and record data for later analysis.
The offline mode is for analyzing historical data generated by the AR system. It provides tools to explore and visualize this data, helping developers understand how the system behaved in the past.
ARGUS’ offline mode comprises three key components: the Data Manager, which helps users organize and filter AR session data; the Spatial View, providing a 3D visualization of spatial interactions in the AR environment; and the Temporal View, which focuses on the temporal progression of actions and objects during AR sessions. These components collectively facilitate comprehensive data analysis and debugging.
“ARGUS is unique in its ability to provide comprehensive real-time monitoring and retrospective analysis of complex multimodal data in the development of systems,” said Silva. “Its integration of spatial and temporal visualization tools sets it apart as a solution for improving intelligent assistive AR systems, offering capabilities not found together in other tools.”
ARGUS is open source and available on GitHub under VIDA-NYU. The work is supported by the DARPA Perceptually-enabled Task Guidance (PTG) program.
ARGUS: Visualization of AI-Assisted Task Guidance in AR